- Decentralized Systems
- May 31, 2023
How AI Enhances Data Consistency in Decentralized Record Systems
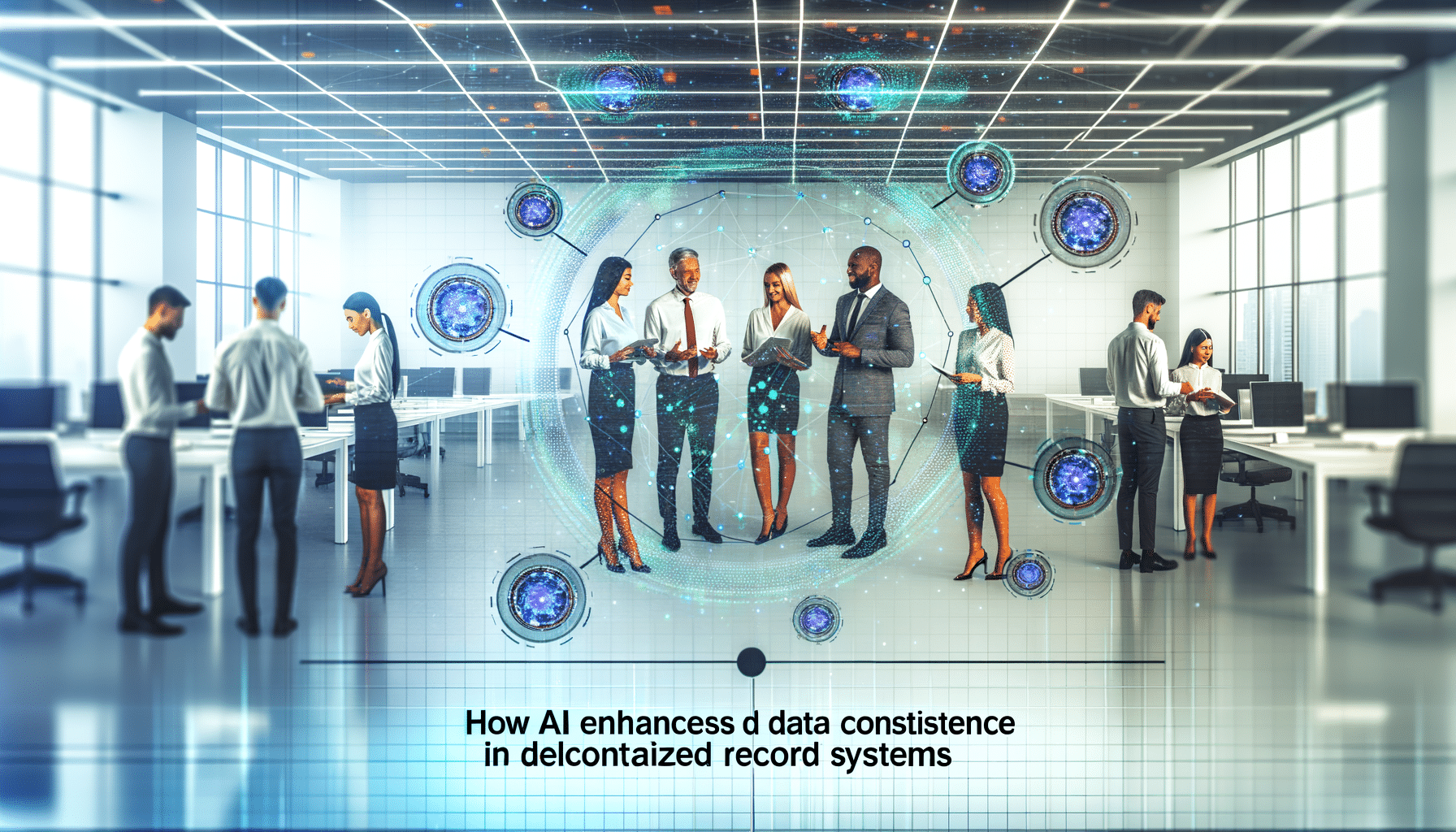
In today’s rapidly evolving digital landscape, maintaining data consistency within decentralized record systems stands as both a necessity and a challenge. The era of centralized data management systems is dwindling, and decentralized networks, empowered by blockchain and AI, are paving the way for future-proof solutions. However, without proper consistency measures, decentralized systems can lead to data silos, fragmented information, and inefficiencies.
The Struggle for Consistency in Decentralized Systems
One of the greatest challenges in decentralized record systems is ensuring data consistency across multiple nodes. Unlike centralized databases where a single point of data truth exists, decentralized systems distribute data across numerous locations, leading to potential inconsistencies. This is where AI plays a transformative role, bringing together advanced analytics, automation, and machine learning to ensure seamless consistency and reliability of data.
AI’s capability to process vast amounts of data swiftly and accurately can effectively eliminate discrepancies, enhancing the reliability of the entire system. But how exactly does AI achieve this in the decentralized setup?
AI as the Pillar for Data Integrity
Leveraging AI, we can harness automated validation techniques that maintain data integrity across decentralized systems. These techniques involve implementing intelligent algorithms that identify and resolve disparities by cross-referencing with recognized data standards.
Think of AI as a diligent supervisor that continually monitors transactions, verifies data accuracy, and reconciles records. It assesses patterns and anomalies in real-time, ensuring that any deviation from the norm is promptly addressed, thus sustaining coherence throughout the blockchain network.
Advanced Algorithms for Data Reconciliation
Data reconciliation represents a significant application of AI in decentralized record systems. Traditional reconciliation methods are often manual, time-consuming, and prone to errors. AI-driven reconciliation automates this process through predictive analytics and machine learning algorithms. These algorithms execute repetitive scrutiny, correlation, and correction tasks at a fraction of the time and cost associated with human involvement.
For instance, in a decentralized record system operated by multiple government departments, discrepancies in citizen data can arise due to varied data acquisition processes. AI can efficiently align this data, reducing redundancy and furnishing an actionable, streamlined dataset.
Ensuring Data Quality with AI-Enhanced Verification
Data quality assurance becomes indispensable in decentralized systems. AI can facilitate this by automating data verification processes, validating entries against pre-defined rules, and highlighting inconsistencies. AI models are adept at handling unstructured data, further refining the verification process and ensuring accurate representation of information.
Moreover, AI enhances data consistency by enabling better error prevention systems. By anticipating potential errors, AI models can deploy corrective measures proactively, minimizing the likelihood of faulty data propagation across the network.
Real-World Implications
The implications of AI-centric data consistency in decentralized systems are manifold, especially for sectors dependent on precise record management like finance, compliance, and legal industries. These sectors heavily rely on data accuracy and security, both of which AI fortifies. By integrating AI, organizations benefit from reduced risks of regulatory non-compliance, enhanced efficiency, and streamlined data governance.
For example, in financial sectors, AI can augment the precision of transaction record-keeping, essential for audit trails and regulatory reporting. This reduces potential discrepancies and builds trust amongst stakeholders through assured transparency.
Conclusion: Harnessing AI for a Consistent Data Strategy
In conclusion, AI’s role in enhancing data consistency across decentralized systems is not merely supportive but fundamental. It reconciles records, ensures data integrity, and fortifies quality control, transforming decentralized networks into robust, reliable systems equipped to meet modern demands. As we advance, integrating AI into our decentralized frameworks not only solidifies consistency but also assures the economic and strategic advantages that come with superior data integrity.
For those navigating the complex arena of decentralized record systems, it is imperative to embrace AI-driven solutions. I’m confident that leveraging AI will revolutionize how we approach data management, allowing us to embrace the vast potential of decentralized architectures with unyielding trust in our data’s accuracy and consistency.
Stay informed about the latest in AI and decentralized systems by connecting with me. The journey of data-driven transformation is just beginning, and I invite you to be a part of it.
Toshendra Sharma is the visionary founder and CEO of RecordsKeeper.AI, spearheading the fusion of AI and blockchain to redefine enterprise record management. With a groundbreaking approach to solving complex business challenges, Toshendra combines deep expertise in blockchain and artificial intelligence with an acute understanding of enterprise compliance and security needs.
Related Posts
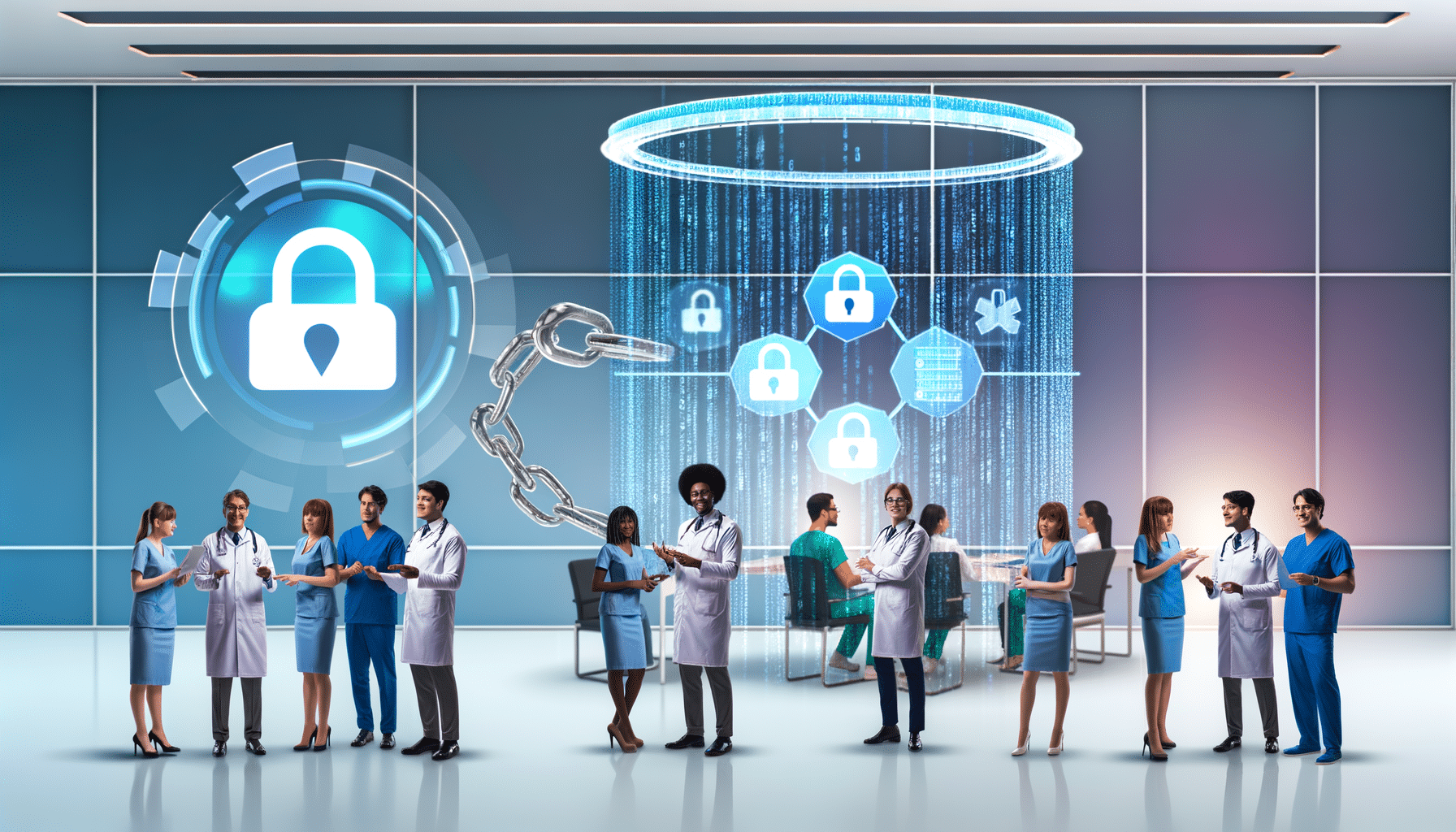
How Decentralized Identifiers (DIDs) Can Revolutionize Patient Record Access
Explore how decentralized identifiers transform patient record accessibility.
- December 8, 2023
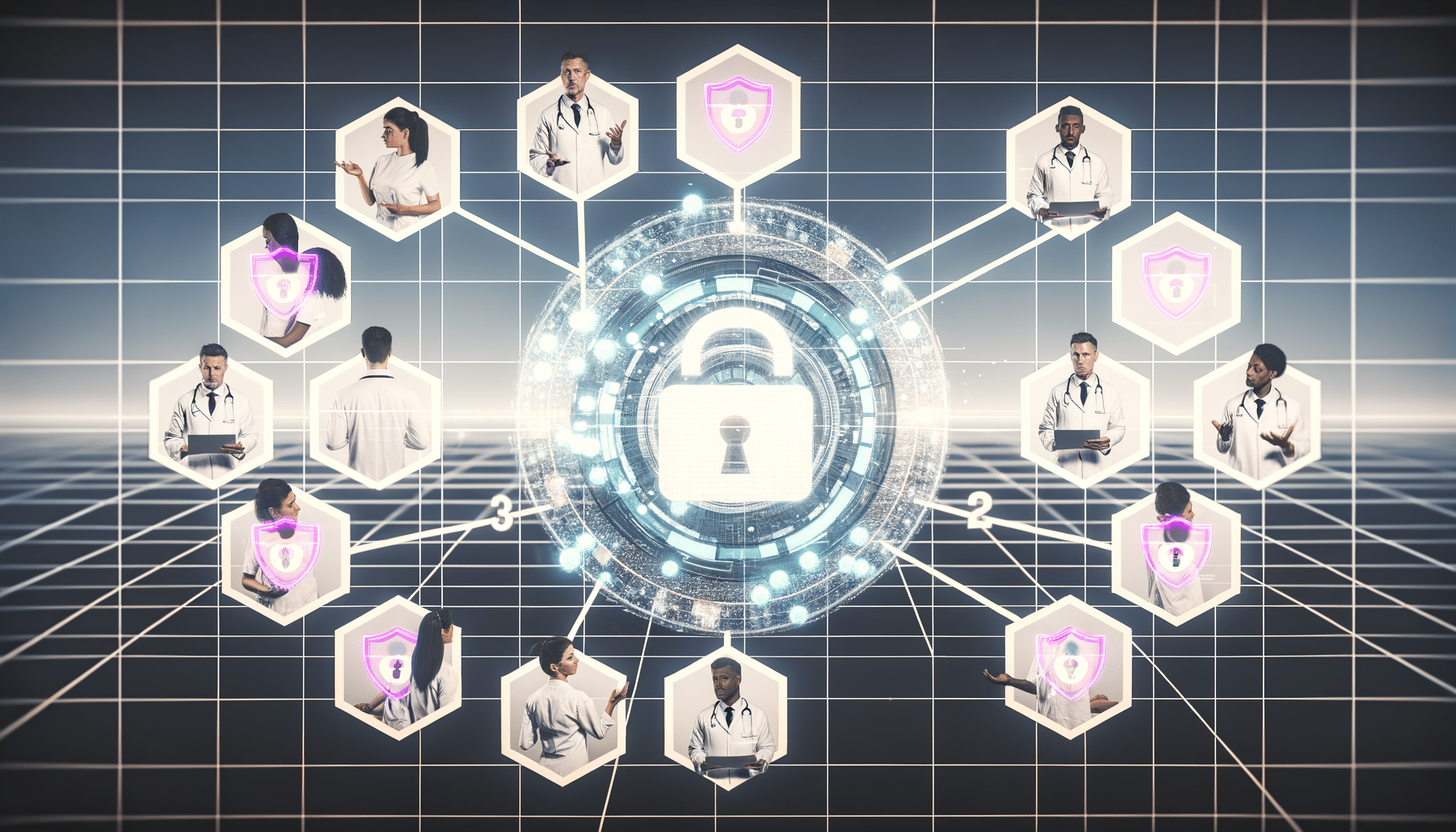
Decentralized Health Data Management: Benefits and Challenges
Explore the pros and cons of decentralized healthcare record management.
- November 6, 2023
Archives
- December 2024
- November 2024
- October 2024
- September 2024
- August 2024
- July 2024
- June 2024
- May 2024
- April 2024
- March 2024
- February 2024
- January 2024
- December 2023
- November 2023
- October 2023
- September 2023
- August 2023
- July 2023
- June 2023
- May 2023
- April 2023
- March 2023
- February 2023
- January 2023
- December 2022
- November 2022
- October 2022
- September 2022
- March 2019
Want to get more content like this?
Signup to directly get this type of content to your inbox!!
Latest Post
Record Organization for Sales Teams
- December 26, 2024
Handling Duplicate Client Records
- December 25, 2024
Quick Reference Systems for Support Staff
- December 24, 2024
Managing Intern Document Handling
- December 23, 2024