- Archive Security
- November 16, 2024
Machine Learning in Fraud Detection and Documentation
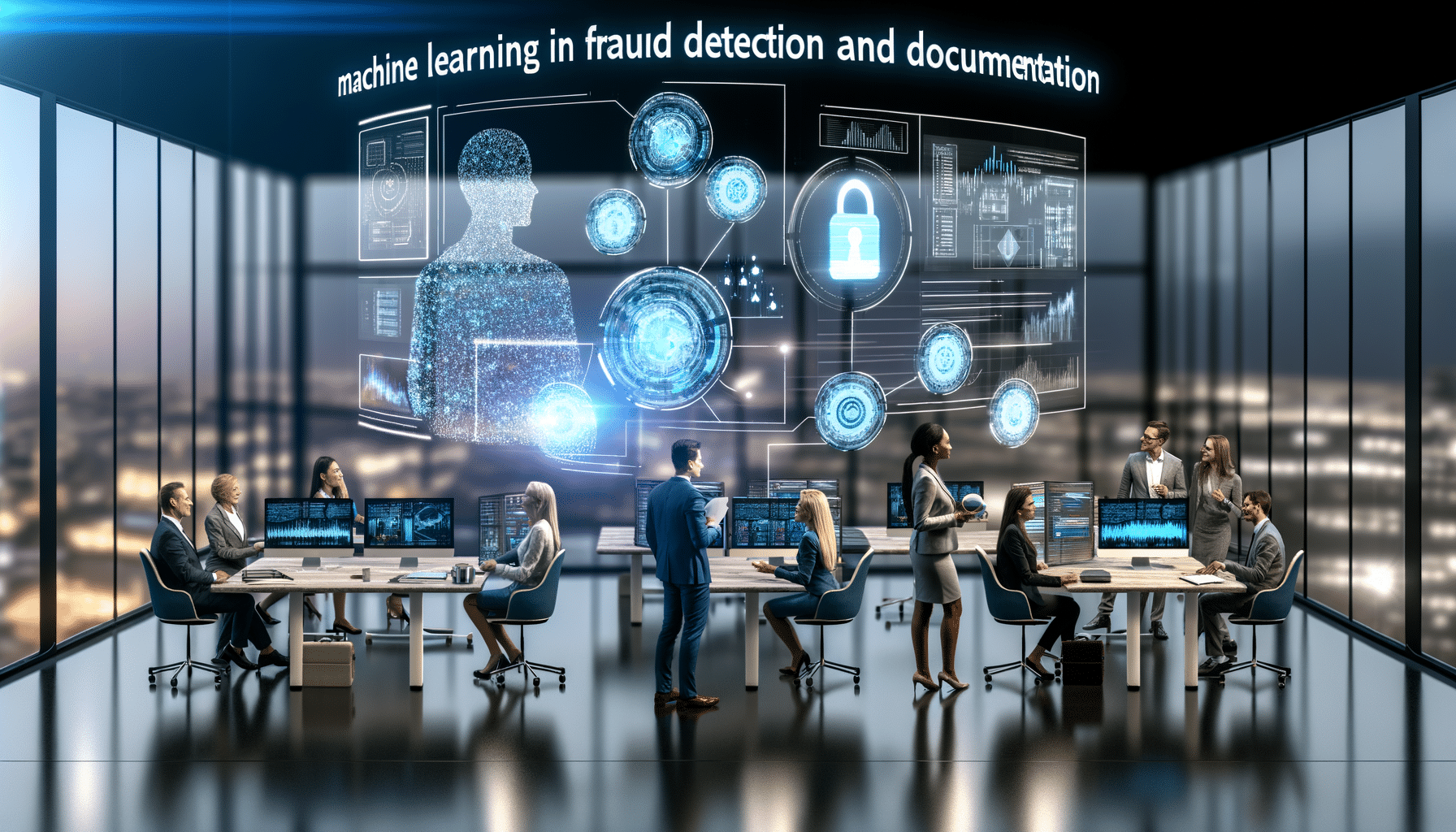
In today’s fast-paced digital landscape, fraud has become a persistent threat in record management, impacting both businesses and government institutions alike. My journey with RecordsKeeper.AI has taught me one fundamental lesson: the future lies in harnessing technology like Machine Learning (ML) to combat these ever-evolving challenges. In this blog, I’ll delve into how ML is redefining fraud detection and documentation, offering strategic insights to participants in the security domain.
Understanding Fraud in the Context of Record Management
Fraud in record management can manifest in various forms, such as unauthorized access to sensitive information, manipulation or alteration of records, and even the creation of fictitious documents. These activities not only put organizational data at risk but can also lead to severe legal implications. The challenge, therefore, is finding efficient ways to preempt such fraudulent actions, and that’s where Machine Learning steps in.
The Role of Machine Learning in Fraud Detection
Machine Learning, at its core, thrives on data. By analyzing patterns and anomalies within this data, ML algorithms can accurately predict suspicious activities before they culminate into an actual fraud attempt. Here’s how it works:
- Anomaly Detection: ML algorithms are excellent at identifying deviations from the norm. By learning from historical data, they can differentiate between normal and abnormal behaviors, flagging potential threats for further investigation.
- Predictive Modelling: With continuous learning from ongoing data inputs, ML models can anticipate fraudulent actions even before they occur, allowing preemptive measures to be adopted.
- Pattern Recognition: Patterns indicative of fraud—like repeated access attempts from different locations in a short span—are recognized swiftly by these algorithms.
These capabilities not only improve detection rates but also reduce the number of false positives that might otherwise overwhelm security teams.
Enhancing Documentation with ML
Documentation represents an integral part of record management, but keeping it accurate, up-to-date, and accessible is a daunting task. Machine Learning assists in optimizing the documentation process in several ways:
- Automated Classification: ML algorithms can intelligently categorize documents based on their content, saving time and minimizing errors that occur with manual processes.
- Enhanced Search Functions: With natural language processing capabilities, ML-powered searches provide users with more relevant results, improving accessibility and efficiency in record retrieval.
- Real-Time Updates: As changes occur within the data, ML can ensure immediate updates, keeping all documentation current and accurate.
These improvements not only streamline operations but also enhance productivity, allowing professionals to focus their efforts on more strategic tasks.
Securing the Future: Implementing ML in Record Management
Adopting Machine Learning in fraud detection and documentation is not just about plugging in a new technology; it’s about transforming the entire approach to record management. Here are some actionable steps for those considering this integration:
- Evaluate Existing Systems: Understand your current record management system’s capabilities and pinpoint areas where ML can offer the most significant advantage.
- Collaborate with Experts: Engage with ML specialists who can customize algorithms to meet your unique requirements, ensuring a solution that’s both effective and scalable.
- Ensure Regulatory Compliance: Always align ML applications with existing industry standards such as GDPR and HIPAA. Compliance is crucial for gaining stakeholder trust and avoiding potential legal repercussions.
Machine Learning is not just a tech buzzword—it’s a future-ready solution that can safeguard your data while enhancing operational efficacy. At RecordsKeeper.AI, we’ve seen firsthand how ML can bolster security measures and improve trust in our systems.
Conclusion
The journey of integrating Machine Learning into fraud detection and documentation is not without its challenges, yet the rewards far outweigh the hurdles. From heightened security and compliance to improved record management efficiency, the benefits are manifold. I encourage you to explore the possibilities with ML, and of course, feel free to reach out if you wish to learn more about how RecordsKeeper.AI can support your organization. Together, let’s embrace this technological frontier and secure a safer future.
Follow me for more insights into how technology continues to transform our world, and stay tuned for further updates from RecordsKeeper.AI!
Toshendra Sharma is the visionary founder and CEO of RecordsKeeper.AI, spearheading the fusion of AI and blockchain to redefine enterprise record management. With a groundbreaking approach to solving complex business challenges, Toshendra combines deep expertise in blockchain and artificial intelligence with an acute understanding of enterprise compliance and security needs.
Related Posts
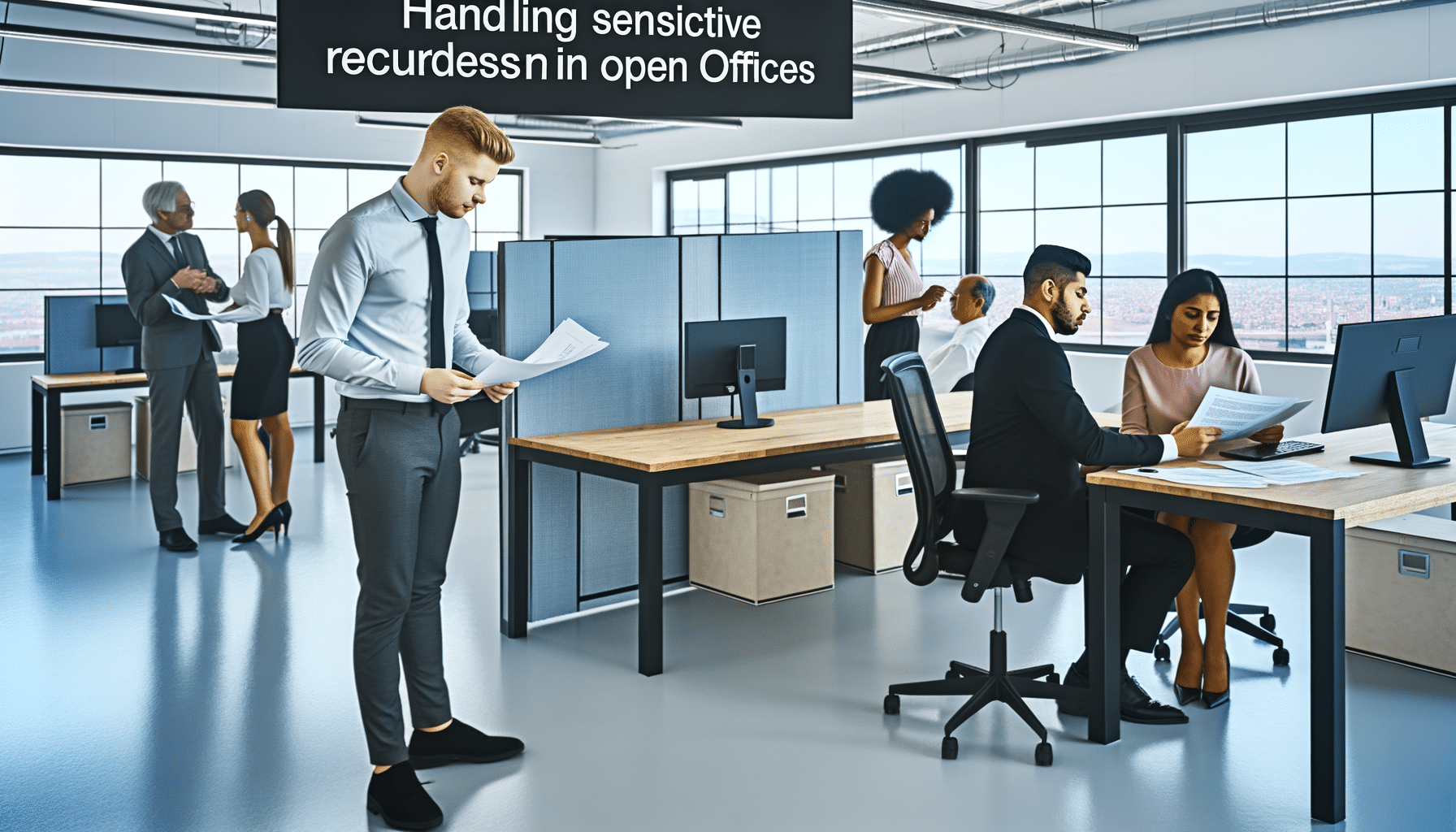
Handling Sensitive Records in Open Offices
Maintaining confidentiality in open workspace layouts.
- December 9, 2024
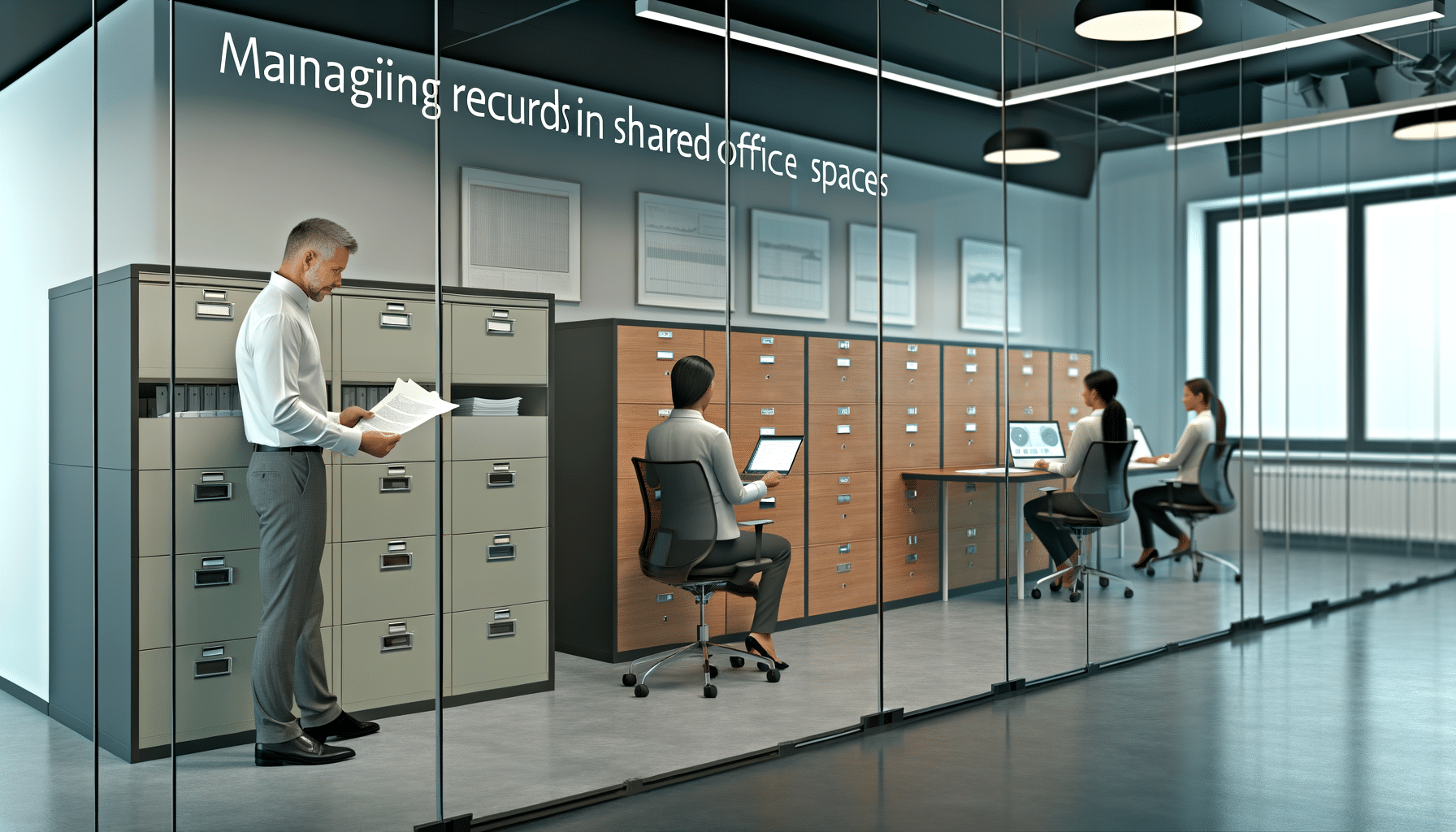
Managing Records in Shared Office Spaces
Keeping records secure in coworking and shared environments.
- November 23, 2024
Archives
- December 2024
- November 2024
- October 2024
- September 2024
- August 2024
- July 2024
- June 2024
- May 2024
- April 2024
- March 2024
- February 2024
- January 2024
- December 2023
- November 2023
- October 2023
- September 2023
- August 2023
- July 2023
- June 2023
- May 2023
- April 2023
- March 2023
- February 2023
- January 2023
- December 2022
- November 2022
- October 2022
- September 2022
- March 2019
Want to get more content like this?
Signup to directly get this type of content to your inbox!!
Latest Post
Organizing External Auditor Access
- December 22, 2024
Document Control in Manufacturing Plants
- December 21, 2024
Handling Rush Financial Report Requests
- December 20, 2024
Managing Record Access After Staff Changes
- December 19, 2024